Age-related macular degeneration (AMD) is a leading cause of severe and irreversible vision loss in older individuals worldwide. It is characterised by the accumulation of sediments under the retina called drusen. In recent years, a distinctive subtype of drusen called reticular pseudodrusen (RPD) has been recognised. It has been shown to be a risk factor for advanced AMD. Interestingly, RPD exist outside the context of AMD. They have been shown to appear in other retinal conditions and can also appear in patients with AMD with no conventional drusen.
Previous studies have identified genetic associations, or risk factors, for AMD. In addition, a few studies have identified genetic associations for RPD. However, these were similar to the ones identified for AMD. We believe that this was a result of these studies investigating patients with predominantly conventional drusen. As RPD can exist outside of the context of pure conventional drusen, we believe there may be a biological difference between them and other types of drusen. We believe they are a distinct disease process.
At the end of 2019, Dr Roy Schwartz from our group was awarded the inaugural EURETINA Retinal Medicine Clinical Research Grant and serves as the principal investigator for the project. The project’s goal is to identify genetic associations unique to RPD by using advanced techniques, including AI, genetic analysis, and big data analysis. Later, the grant inspired other groups to build on this project, and our Australian collaborators led by Prof. Robyn Guymer secured an additional, larger grant to tackle this important problem further.
To answer these questions, our project uses the UK Biobank, a large-scale biomedical database and research resource containing genetic, lifestyle and health information from half a million UK participants. It includes genetic data of the 500,000 volunteer participants, as well as retinal imaging of 85,000 participants. So, naturally, we would need to identify those participants with RPD and analyse their genetic code to identify relevant associations. The problem: Each participant has 128 OCT scan slices (called B-scans) per eye, meaning that we would need to examine almost 22 million scans to find those with RPD!
Since this task would have taken many human hours (or even years), Dr Schwartz developed a deep learning model. The model has several steps as part of a machine learning pipeline. First, it identifies poor quality scans, which cannot be used with certainty to identify RPD. It then identifies drusen (of any type) in the remaining scans. Finally, among scans with drusen, the model can segment (or colour the areas of) conventional drusen and RPD separately. This allows us to get a measure of the area of RPD and drusen for each patient, an essential parameter for genetic analysis.
Below is a sneak preview of the model’s output. In blue are conventional drusen and in green RPD. We are very proud to say that this is the first model to use deep learning to detect these lesions separately with high accuracy and the first to allow accurate quantification of their load in the eye.
Be sure to sign up for our newsletter for further updates on this project, including a publication coming soon!
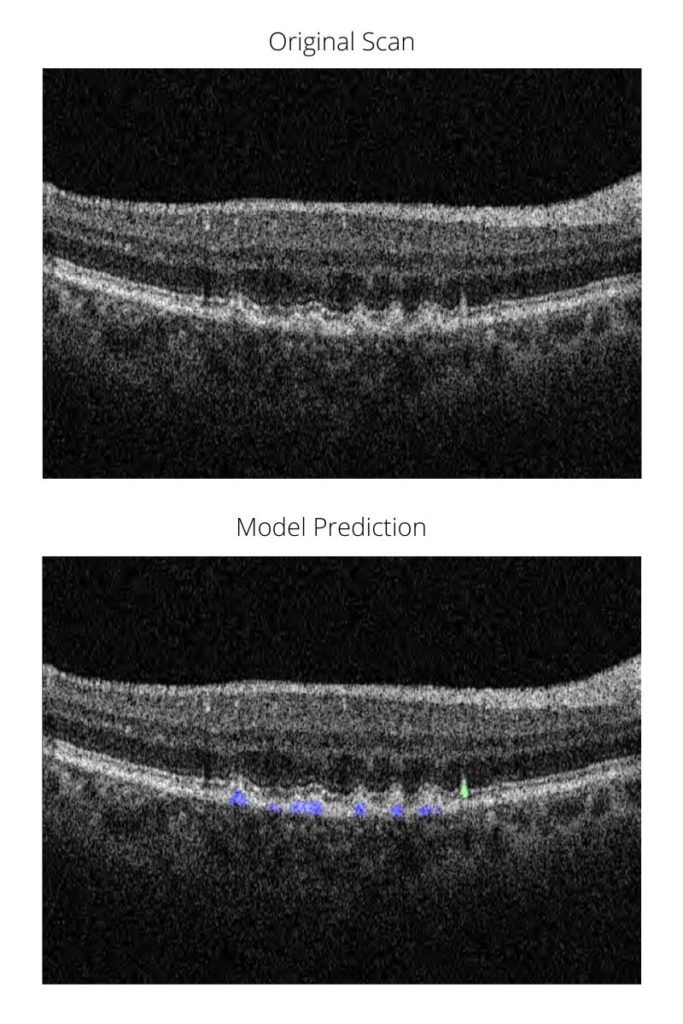